In 2020, the economy is once again teetering on the edge of what some are calling a fiscal cliff.
In the midst of COVID-19, a global economic crisis is threatening the livelihoods of small business owners everywhere. In ordinary times, 50 percent of small businesses go out of business in the first 5 years. In today’s extraordinary times, nearly 7.5 million (~25 percent) of small businesses in the U.S. alone have been at risk of closing permanently in a matter of months (Source: Main Street America’s Small Business Survey 2020).
Among the all-time top contributing factors to small business survival? Cash flow management and access to capital. Whether in the midst of a pandemic or a devastating life event, business owners are more likely to successfully make it through tumultuous times if they can:
- Efficiently manage the inflow and outflow of cash
- Understand their cash position to qualify for government aid and/or loans with flexible borrowing terms
- Use financial insights to map out revenue and spending scenarios
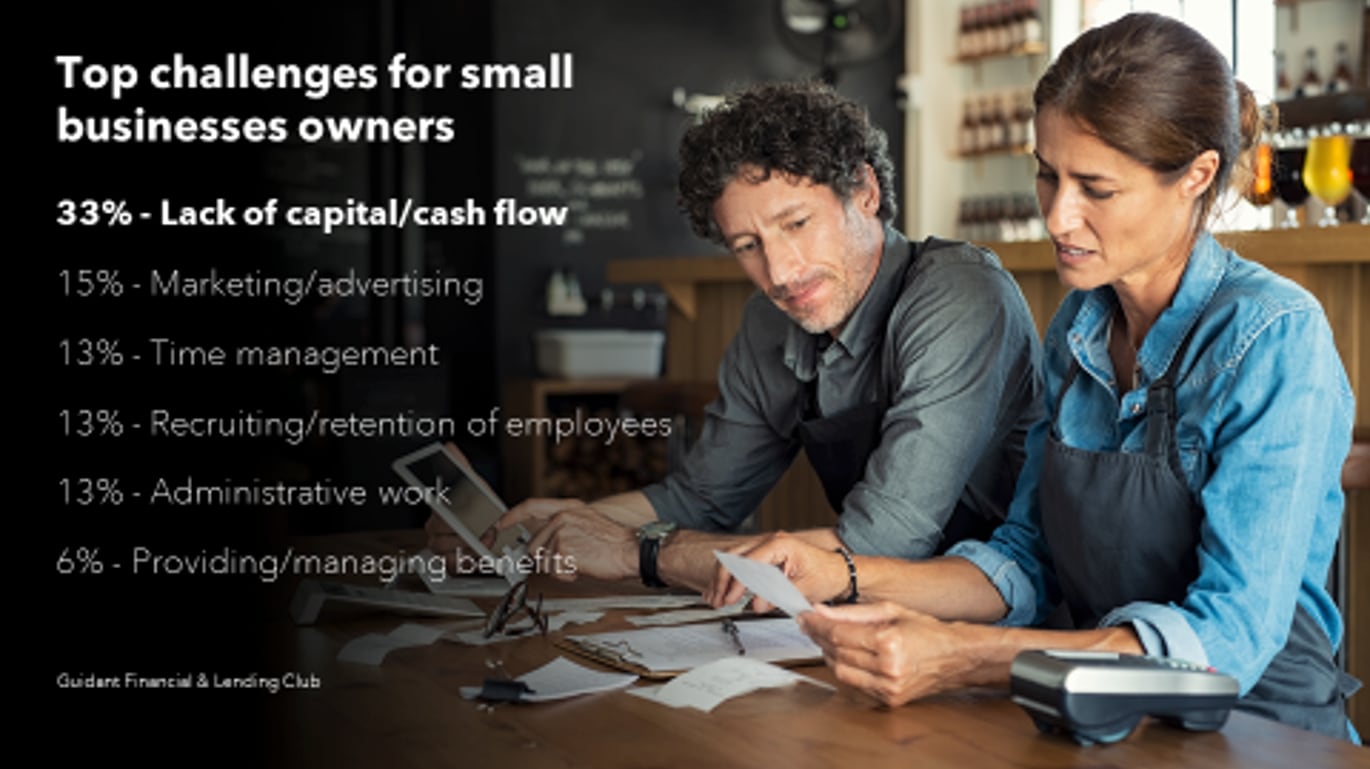
To that end, our company immediately took steps at the outset of the pandemic to help consumers and small businesses access desperately-needed capital through four key initiatives and AI-driven innovations, such as Intuit Aid Assist and the QuickBooks Capital for Paycheck Protection Program.
As part of Intuit’s Small Business and Self-Employed Group, it’s my team’s responsibility to apply artificial intelligence (AI) and large-scale machine learning (ML) to help small business owners build financial resilience in the face of economic fluctuations.
Sadly, as data scientists we can no longer look at global economic crises as anomalies. Just a dozen years ago, we witnessed the worst economic disaster since the Great Depression of 1929 with the Great Recession of 2008. And, the World Bank expects the pandemic to plunge most countries into recession in 2020.
The frequency of such crises has implications for AI and machine learning itself. Meaning, we can’t just remove them from our models, smooth over the results, and assume that business as usual will prevail. We have to factor these events into our work, building them into a more realistic approach.
With that in mind, my AI/ML team has been working with Intuit development teams to create a cash flow planner to help our customers make better decisions about money-in and money-out by analyzing their historical patterns to project what’s likely to happen. In this blog, I’ll offer a glimpse into that work, the results we’ve achieved so far, and the work still to come.
Solving the cash flow puzzle
When we first began work on our QuickBooks Online Cash Flow planner, we had a simple goal to enable our small business and self-employed customers to see their current cash balance, understand where it came from, and project it forward.
Turns out it wasn’t so simple.
When you look at the money coming in and going out of a business, the patterns can be idiosyncratic and vary dramatically over time. Some cash streams are periodic. Some are random. Some are never repeated. Some streams are actually aggregates of other individual streams. And we’re not just talking about cash. There are customer invoices payable at uncertain times, bills due on different schedules, and dependencies among income, taxes, sales, and payroll.
All of which needs to be modeled out.
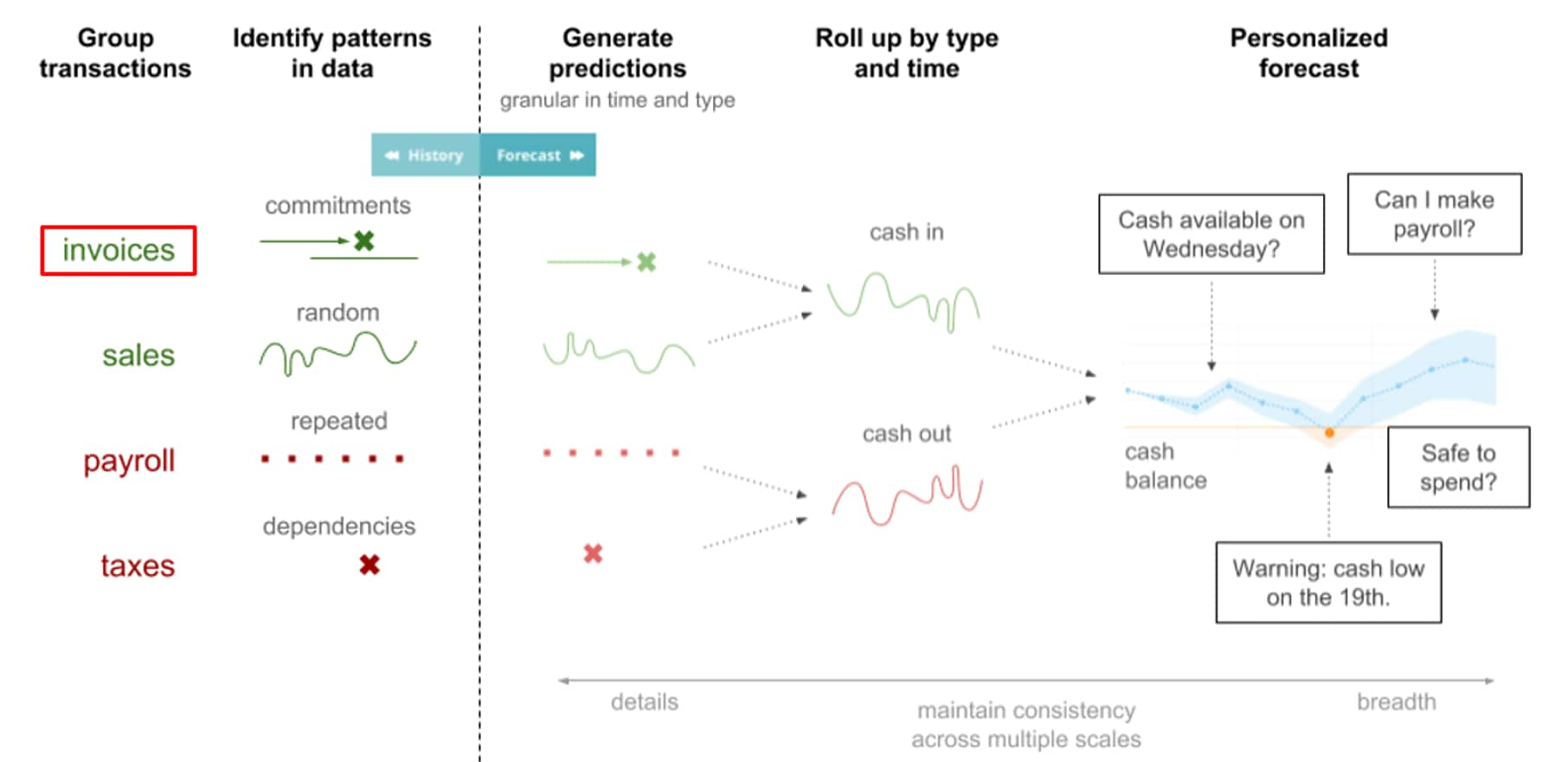
QuickBooks invoice prediction in action
So instead of simply aggregating a bunch of time series data points together to build a forecast, we discovered that we’d need to construct individual models for every single transaction stream, identify the patterns in each of those streams, build models that generate predictions, and aggregate those predictions by type and time. Only then would we be able to answer a question like, “Will I be able to make payroll this week?” or “Can I spend this money today?” or “Is my cash about to run low?”
Pivoting quickly to support small businesses
At this distressing time for small businesses all over the world, our AI/ML team looked closely at whether we were building the righ models and solving the right problems within Intuit’s QuickBooks Online Cash Flow planner to address our customers’ most pressing financial challenges.
“If the virus doesn’t kill me, the stress is gonna kill me. You’re afraid for your physical health, you’re afraid for your financial health.”
“My entire industry has had the rug pulled out from under it.”
“Most of us don’t really have a lot of cash on hand to survive something like this where you have no revenue coming in.”
Invoices: turning payments due into cash
A key aspect of the larger cash flow forecasting problem is helping small businesses predict when payments due will turn into cash. We view this as a two-stage question. First, will this customer invoice actually be paid? Secondly, when will it be paid? From QuickBooks Online data, we noticed the tendency for small businesses that generate a lot of invoices to struggle with getting paid on time. Some customers pay late and others require extensive follow-up to pay at all.
To address the first stage of the problem, we built a classification model to predict whether a given invoice is likely to be paid. We based it on a standard gradient boosted tree model with class weight tuning to address label imbalance. For the timing question, we needed to be able to predict when customers were likely to pay.
This took a certain amount of nuance. We didn’t want to err on the side of false positives, which could result in time-consuming follow-up with customers who were likely to pay anyway, and inadvertently eroding the trusted relationship between the business and its customers. On the other hand, we didn’t want to let invoices linger any longer than necessary.
So, to address the second stage of the problem, we used gradient boosted trees with quantile loss to model different confidence levels for the difference between the due date and the payment date. This way, we were able to train, test, and validate the model using the company’s own temporal data, build company and client temporal features, and apply the appropriate label to each invoice.
Here’s an example of what the final result could look like for a QuickBooks Online business owner.
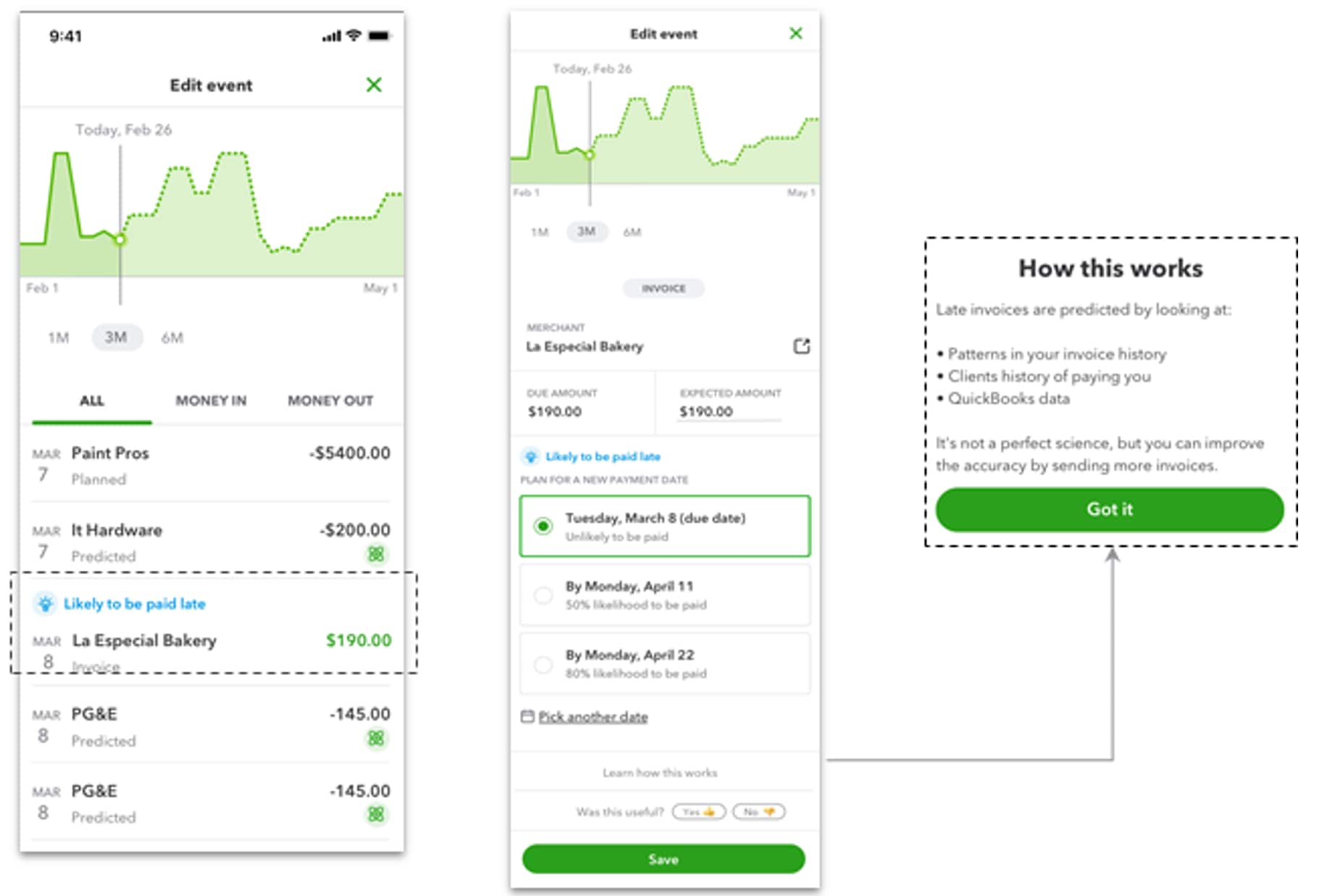
QuickBooks Online invoice prediction in action
As you can see in this illustration, when they go into the Cash Flow planner to review a forecast of transactions, a light bulb will flag any invoices that are “likely to be paid late.” When they click on it, the original due date and two possible payment dates will appear—one with a 50 percent likelihood of being paid on time, and another with an 80 percent likelihood of being paid. By offering a range of confidence intervals, we instill confidence with our customers that they can act on the information we’ve provided to better predict their cash flow. Moreover, we enable them to understand how we’ve arrived at the recommendation. In customer interviews, we learned that “showing our work” was an important feature.
But, the invoice problem is just one challenge our AI/ML team is tackling for our customers.
Modeling small business sales trends
When we launched Intuit’s QuickBooks Online Cash Flow planner in January 2020, we couldn’t have imagined what was ahead for small businesses around the world, in just a few short weeks.
With the onset of COVID-19 in March, we witnessed a precipitous decline in our customers’ small business sales. Many service businesses experienced a 100 percent drop in sales (e.g., beauty salons), while some product-focused businesses were able to gradually transition to online sales models.
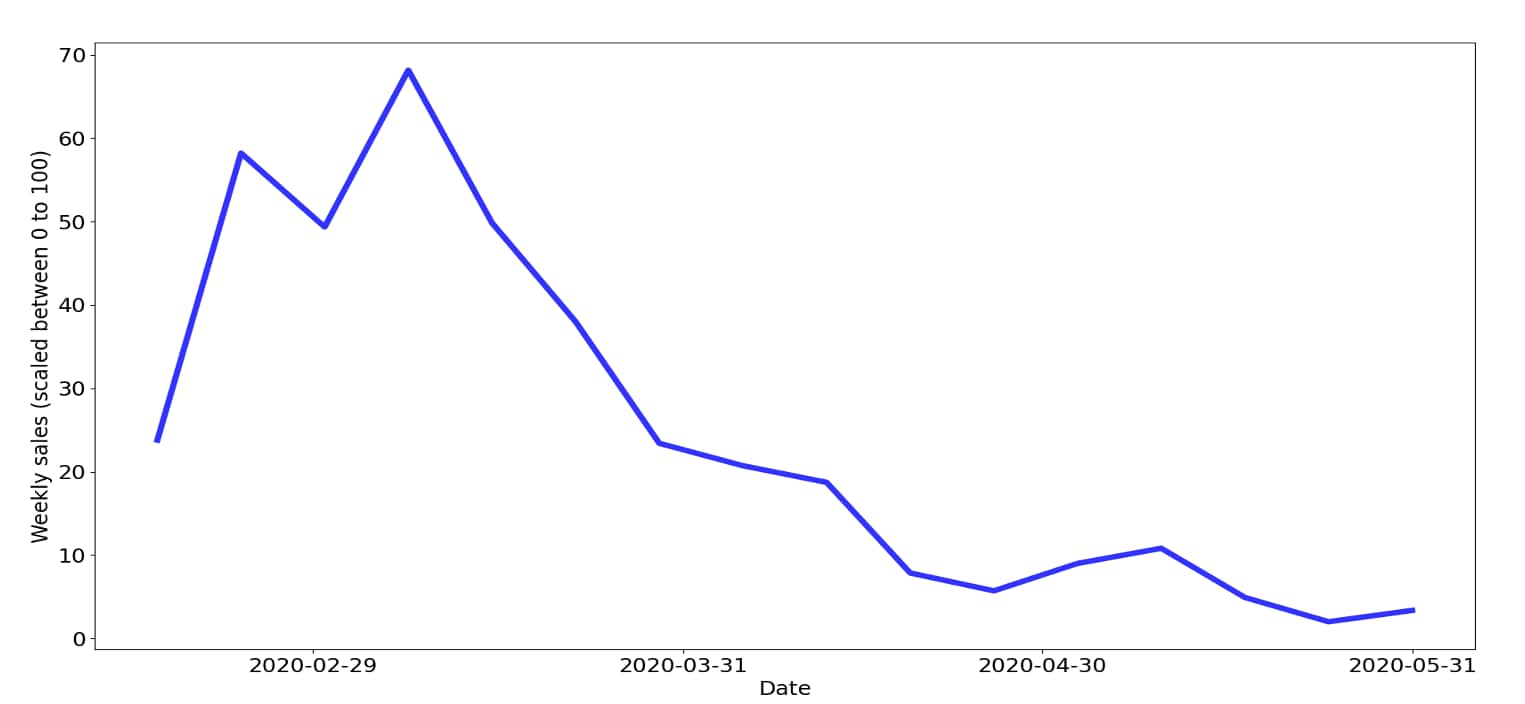
Small business sales have been significantly impacted by COVID-19
[Representative Mean of Weekly Sales for a sample companies selected
from major cities across industrial sectors hit by the pandemic.]
Before the global pandemic, our AI/ML team built models for small business sales, taking into account businesses with a steady stream of sales, as well as those with little data or sporadic sales patterns. For example, an ice cream shop with a high volume of sales throughout the year, or a Halloween shop with seasonal sales that peak annually and account for the bulk of the business.
In the first scenario, it was relatively straightforward to build a time series model, working with a rich data set from a predictable sales curve. In contrast, the second scenario was much more difficult to model, due to sparse data from sporadic sales patterns or seasonal sales peaks.
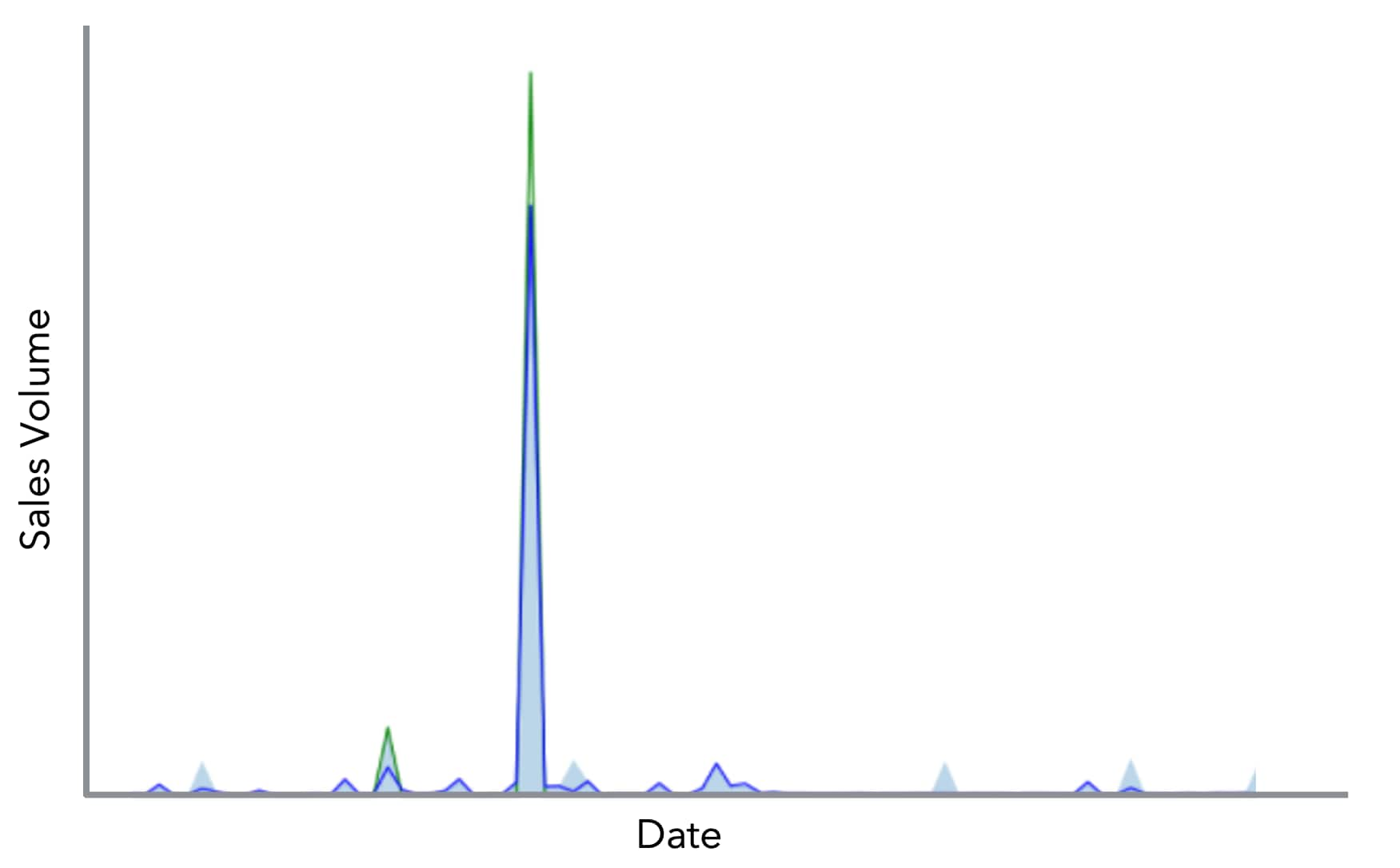
Modeling sparse data with hurdle models
So, how did we reconcile these two worlds? We used hurdle models, which can handle “zero count” events when no business is coming in (e.g., holidays, certain days when a business is closed, seasonal businesses only open during certain months of the year). This approach gives us an extra level of flexibility for smoothing out the data to effectively support cash flow planning scenarios for a range of business models.
But, as we’ve discovered all too well—and especially in the times of COVID-19—we no longer live in a world in which we can treat economic crises as once-in-a-generation events. What were anomalies in the past now need to be factored into our approach to AI/ML models.
So, that’s what we’re doing. Dealing with data from the past few months to model this out. We’re exploring new algorithms that can handle sudden shifts and changes to help small business owners be able to say, “Okay, I’m going through this really tough time, and need to understand what my sales and revenue will look like in the future. I know that the rate and volume of sales won’t be where it was before, but can my business survive through the end of the year as the economy begins to open back up?”
As data scientists here at Intuit, what we find most satisfying is tackling really big problems that make a difference in the everyday lives of people. That’s why our AI/ML team has been so pleased to hear from customers that our cash flow planner is doing just that—and why we’re so committed to seeing them through to a better day.
“It’s critical, because it lets us know how we’re doing, are we financially healthy, if the next few months are going to be difficult, if there are purchases that don’t need to be made.”
“It helped me tremendously to be able to juggle expenses with people. I was able to not have to lay off anybody, and it helped us to identify which people would be willing to stretch my payroll until orders get placed again.”
What’s next?
As you can see in this blog, the cash flow problem is incredibly complex. When we set out on this innovation journey, we built our models for the US population. During the pandemic, we opened up the cash flow planner to reach a broader audience of small business customers in the UK and Australia, where we also serve small businesses with QuickBooks Online.
There are so many different types of small business circumstances to take into consideration—and distinct cash flow streams requiring finely-tuned individual models—each with the possibility of making a huge difference in the financial health of small business owners.
The same is true for consumers, like you and me.
In the future, we’ve set our sights on expanding the cash flow planner from small businesses to consumers. With access to intuitive AI-driven personal finance tools, more people will have the information they need to better manage the ebbs and flows of their cash flow. Even better, they’ll be untethered from the tedium of manual spreadsheets for greater efficiency and accuracy.